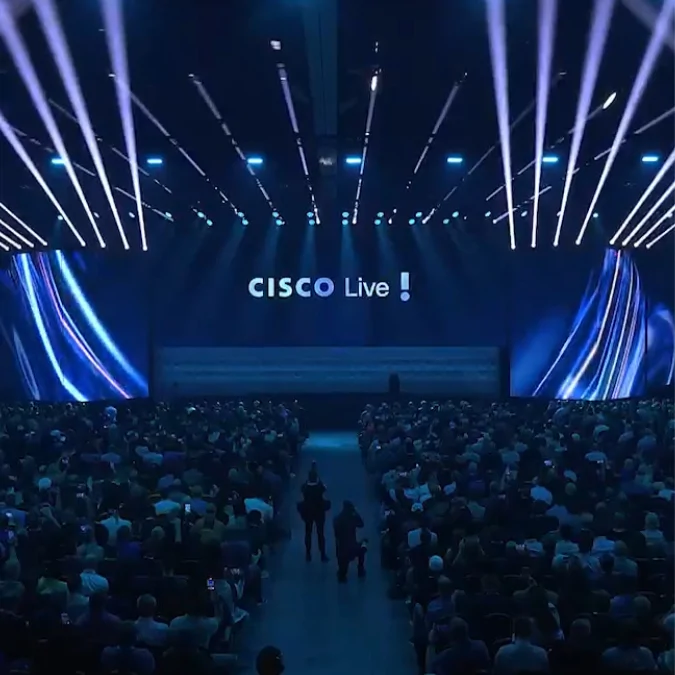
Explainable AI explained
14 Jul, 20234 minsDevelopments in Artificial Intelligence (AI) have prompted policy debates as to the practica...
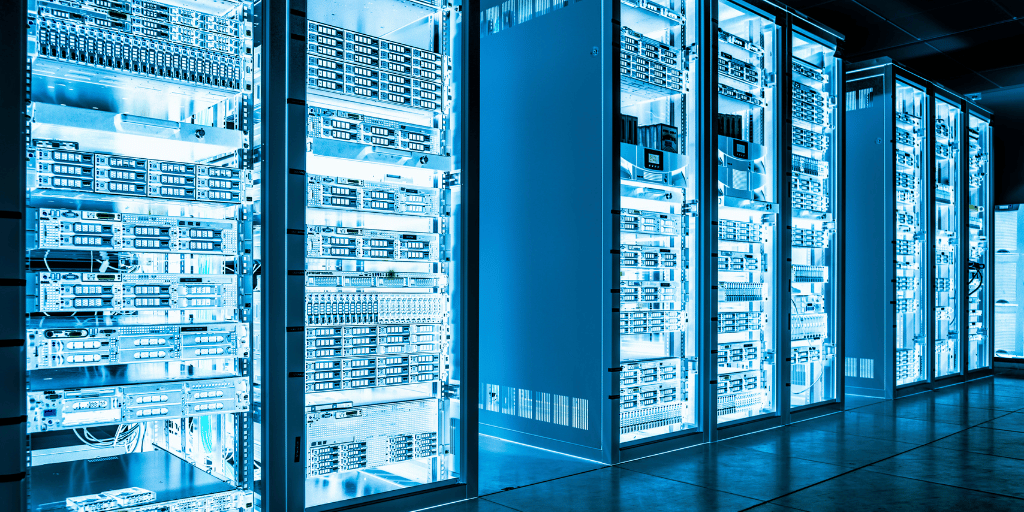
Developments in Artificial Intelligence (AI) have prompted policy debates as to the practical and ethical implications of the technology.
Included in this, are increasing calls for some form of AI ‘explainability’, as part of efforts to embed ethical principles into the design and deployment of AI-enabled systems.
In the UK, for example, calls have come from the House of Lords AI Committee, which argued that “the development of intelligible AI systems is a fundamental necessity if AI is to become an integral and trusted tool in our society.”
In other words, organisations and individuals need to be able to understand how AI systems work, in order to ‘buy-in’ to the process and be fully committed to integrating them. As humans, if we can’t see or understand the value of something, we are unlikely to commit time and energy to it. It needs to be made less abstract and more tangible.
So, what is explainable AI?
Explainable AI (XAI), also known as Interpretable AI or Explainable Machine Learning, is a set of processes and methods that allows users to understand the reasoning behind decisions or predictions made by AI’s machine learning (ML) algorithms.
This ‘explainability’ aspect will be crucial to garner the trust and confidence needed in the marketplace to prompt broad AI adoption. And companies are clearly beginning to jump on board – the global explainable AI market size is estimated to grow from $3.5 billion in 2020 to $21 billion by 2030.
Why is explainable AI needed?
According to The Royal Society of scientists, there are many reasons why some form of interpretability in AI systems might be desirable or necessary.
These include:
- giving users confidence that an AI system works well.
- safeguarding against bias; adhering to regulatory standards or policy requirements.
- helping developers understand why a system works a certain way, assess its vulnerabilities, or verify its outputs.
- or meeting society’s expectations about how individuals are afforded agency in a decision-making process.
As with many AI scenarios, there needs to be ‘approval’ from the whole team in order for it to be helpful. Think of it like incorporating a new filing system at work – if people don’t understand how it works or why it’s beneficial to their role, they likely won’t use it properly, or at all, rendering it useless
Establishing trust and confidence in AI impacts the speed of its adoption which in turn determines how quickly and widely its benefits can be realised. XAI is a crucial component for growing, winning, and maintaining that trust in automated systems. Without trust, AI won’t be fully embraced.
What is it about AI that makes transparency so important?
Many of today’s AI and ML tools are able to produce highly accurate results, but are also highly complicated, if not opaque, rendering their workings and outputs difficult to interpret. These ‘black box’ models can be too complicated for even expert users to fully understand.
Shining a light on the data, models, and processes allows operators and users to gain insight and observability into these systems. Not only does ‘explainability’ allow for optimisation, but it also enables users to more easily identify and mitigate any flaws, biases, and risks.
Frameworks that allow domain experts to satisfy any scepticism by digging deeper, examining each layer, will help to them to establish trust in the system, as the user increases their own productivity and learning.
By pulling back the curtain on the inner working of data systems, risks can be reduced – a highly important ability to have within automated systems. The importance is clear when considering areas such as bias in AI systems. In reality, real-world data is messy, it will contain missing entries, can be skewed or subject to sampling errors. There have been several high-profile instances of image recognition systems failing to work accurately for users from minority ethnic groups, for example.
Research in explainable AI is advancing, with a diversity of approaches emerging. The extent to which these approaches are useful will depend on the nature and type of explanation that is required.
Explainable AI enables IT leaders to better understand and leverage the insights that AI offers and will be a gamechanger for businesses’ AI strategies. And, as interest in the process increases, as will the need for talent with the skillsets necessary to implement such systems.
Looking to take your first steps in the tech sector or searching for your next role? Get in touch with our expert team today.